4 min read
Expression Vector Optimization for Protein Production
Dec 21, 2019 3:42:20 PM
By:
Jean Peccoud
Dec 21, 2019 3:42:20 PM
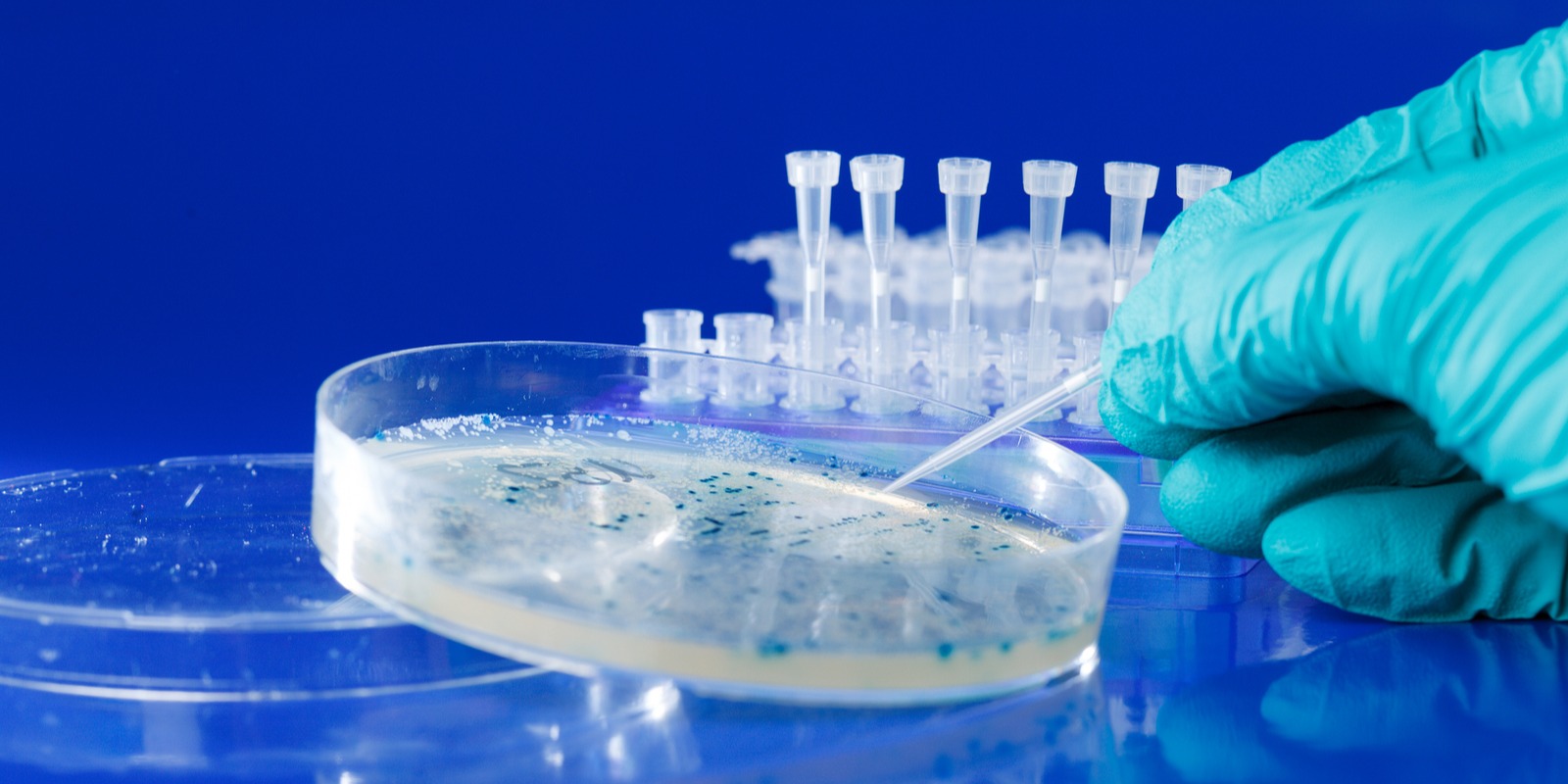
Most recombinant proteins can be produced by simply cloning the corresponding genes into standard expression vectors. With increased speed and reduced cost, this process is simple and efficient. However, it is unlikely to lead to optimal levels of recombinant protein expression. The goal of expression vector optimization is to maximize the yield of a recombinant protein manufacturing process.
Problem
Several control parameters (transcription, translation, and plasmid copy number) can be leveraged to tune protein expression in E. coli. When trying to maximize protein expression, a naïve mistake is to try upregulating all parameters at the same time. An expression vector design strategy relying on a high plasmid copy number, a strong promoter, and an efficient ribosome binding site will not lead to great results. There will likely be a fitness penalty for the host cell, affecting cell growth. The recombinant protein may misfold and accumulate in inclusion bodies. Ultimately, the yield of the desired protein will most likely be poor.
Optimal protein expression requires a careful balance of transcription, translation, and plasmid copy number to maximize the production of a recombinant protein. Unfortunately, it is not possible to predict which combination of genetic parts will make the best expression vector.
Instead, the best expression vector for a gene can come from mixing and matching plasmid components. It is possible to produce a family of related plasmids by combining different genetic parts using recombinant DNA technology. Using this plasmid library, it is then possible to collect enough data to show how these different parameters interact with each other. These data can be combined in a mathematical model that will be used to predict the optimal combination of genetic parts for each gene.
As expected, a large set of expression vectors leads to a large dataset and strong predictions. In this context, being able to design and test the entire library of expression vectors with high accuracy and reproducibility determines the overall success of the experiment.
Solution
Using the GenoFAB software, we helped the client develop a process encompassing all phases of the project.
Design: Combinatorial library of 48 expression vectors
First, GenoFAB helped the team design the optimal minimal expression vector. This vector design would facilitate assembly operations. It also included various tools to finely adjust different expression control parameters.
The team generated a library of 48 plasmid sequences using the plasmid design services to perform a proof of concept experiment.
Build: combinatorial assembly of the plasmid library
Minimizing the cost of assembling the plasmid library was a priority. To this effect, GenoFAB helped the team design an economic strategy to reuse synthetic DNA fragments. Using Build services, they produced 96 PCR primers which added common overlap sequences to the ends of each gene. They could then use Gibson assembly and other standard molecular biology techniques to create the plasmids.
After that, we designed a plasmid assembly process optimized for speed, stability, and reproducibility. To facilitate preparation of assembly reactions, the process generated pipetting instructions for their liquid handling system. We directly derived pipetting schemes to prepare PCR and Gibson assembly reactions from Laboratory Information Management System (LIMS) data.
Further, The LIMS supported the process allowing users to generate barcoded labels to track samples throughout the entire workflow. The LIMS data model was customized to monitor all samples generated by the vector assembly process.
Finally, the plasmid assembly workflow ended with a sequence verification step. Short sequencing reads were assembled using a de novo assembly strategy. The full sequence of each assembled plasmid was verified by the reference theoretical DNA sequence.
Test: automating the estimation of gene expression data
One of the potential bottlenecks of this project was the testing phase. It can be challenging to collect recombinant protein expression data on a large number of expression vectors. It would have been too slow and expensive to grow each cell line in a fermenter and purify the protein.
Instead, the team decided to use the expression of selection markers as a proxy. With this strategy, they used a much simpler measurement: cell line fitness in different growth conditions.
This strategy permitted using a high throughout plate imager to measure gene expression. GenoFAB developed a way to import, process, and store all raw images in a database. We also developed a data service to then analyze the data. The application aggregated gene expression data and LIMS data to estimate protein expression levels achieved by each of the 48 plasmids.
Learn
Lastly, a statistical model made it possible to describe how the vector architecture impacted gene expression. This helped determine how the different parameters influenced the yield of recombinant protein production. The model made it possible to tune the plasmid copy number, the transcription of the gene of interest, and its translation to maximize gene expression.
Results
All in all, results from this experiment led to a follow-up project to design a next-generation expression vector. For this project, the client considers patenting the expression vectors to gain a competitive advantage in the marketplace.
Need help?
Schedule a call to discuss your research informatics needs. Our team of experts can help you choose the GenoFAB plan that best matches your needs.
Recent Posts
8 steps to increase the productivity of your lab
We all wish to increase our lab productivity. We have deadlines to meet, publications to write, and...
Using high-throughput processes to optimize protein expression
Scientists in charge of protein expression optimization projects all too often fly by the seat of...
Vero cells, the green monkey kidneys cells used to produce vaccines
Vero cells are commonly used to study viruses, treatments, and vaccines. The reason for this lies...